In our series “Motee Minute”, we let our Motees have their say on the latest tech trends – unfiltered, frank, and opinionated. This week we’re hearing from Alberto. Alberto is a Solutions Architect and a Senior Project Owner at Motius. His main area of expertise is Artificial Intelligence (AI) and Data Science applications. Before joining Motius, Alberto worked at the Audi AI Lab in autonomous driving applications. Before that, he got a Ph.D. in machine learning for swarm robotics while working at the German Aerospace Center (DLR).
I am often asked: what is FunKI? The answer is fairly simple:
FunKI = AI + 5G
It sounds easy enough, right? It’s not so simple and I will explain to you why in this blog post. So, let’s get started into FunKI by first introducing AI and 5G technologies.
The Thing About AI
We’ve heard it a hundred times: AI is that evil entity that is going to rule us all, take our jobs, and control our every move. We buy what it tells us to, and pick our friends according to its will. But on the flip side, we see headlines where AI is painted as the good entity that is going to improve our lives, expand humans’ capabilities, and let us retire before we even start working.
But is AI good or evil?
..And the Thing About 5G
5G stands for the 5th generation mobile network. Some shout that 5G is evil, that it is bad for our health and will permit Bill Gates to monitor and control us through radiation waves beaming from our devices. Other people however whisper that 5G is a technology that is designed to connect virtually everyone and everything with each other, including machines, objects, and devices. Imagine billions of connected devices gathering and sharing information in real-time resulting in reducing road accidents; life-saving applications thanks to lag-free guaranteed connections; or production lines so predictive they can prevent interruptions long before they occur.
So: Is 5G good or evil?
As you might expect, we at Motius are on the good side of it all. We are technology lovers and strongly believe in the potential benefits that AI and 5G could bring as individual technologies. So, imagine what we could achieve if we mix them together into one glorious project. THAT is FunKI!
“What a combo! AI and 5G. This is what FunKI is all about!”
Why Does 5G Need AI?
Communication functions are typically described by the Open Systems Interconnection model (OSI model). The OSI model partitions the flow of data in a communication system into seven abstraction layers, from the physical implementation of transmitting bits across a communications medium to the highest-level representation of data of a distributed application.
AI techniques are already being used successfully at the higher protocol layers of 5G systems. In contrast, traditional mathematical-abstract models based on idealized assumptions have only been used in the first two layers; namely the physical layer (PHY) and media access (MAC) sublayer.
The sometimes-contradictory requirements in terms of reliability, transmission rate, number of terminals per area, latency, available radio resources, energy efficiency, complexity, and hardware costs are making implementation with traditional methods increasingly difficult. If, for example, the mathematical modeling of the problem is no longer possible (model deficit) or leads to a very complex model (algorithm deficit), data-driven machine learning (ML) methods represent a promising approach for gaining an understanding of the model, as well as for system and technology design.
”In FunKI, we are investigating how 5G can benefit from data-driven machine learning methods.”
FunKI (in German: Funkkommunikation mit Künstlicher Intelligenz) is a research project funded by the German Federal Ministry of Education and Research (BMBF). FunKI brings together 8 pioneering teams, connecting companies (namely Intel, Nokia, Creonic, and, of course, Motius) and researchers (namely DFKI, and Universities of Bremen, Stuttgart, and Kaiserslautern) in the fields of 5G wireless communications, Artificial Intelligence and microelectronic system design to address the challenges ahead in the implementation of future communications systems.
In FunKI, Motius is benefiting from broad know-how acquired in past projects in the field of AI and wireless communications technology. We are using this expertise to investigate some key enabler technologies for 5G. Let’s now get a little bit more technical to dive deeper into FunKI together with the Motius team that’s working on this project.
The Motius FunKI Team
Our team is composed of four engineers in the fields of machine learning and wireless communications, plus a project manager (my humble self). Let’s dive into the topics we are focusing on in FunKI.
Improvement of the Pseudo Random Access Channel (PRACH) detection. This is required to initialize the connection of mobile users to a cell tower. In particular, we are investigating the combination of signals from multiple antennas using different neural network architectures. This work will allow 5G networks to service more users, as well as to eliminate dead zones in rural areas.
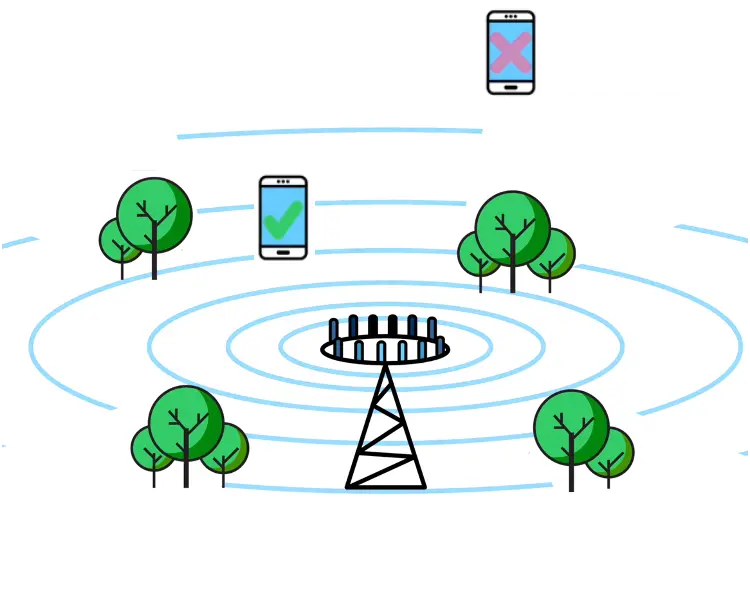
Channel estimation using Deep Learning. That is, given a received signal, estimate the effects of the communication channel on the transmitted signal. For that, we are investigating how to profit from convolutional (CNN) and recurrent neural network (RNN) architectures. In particular, we use basic CNNs, as well as image reconstruction architectures to carry out denoising and super-resolution tasks. Regarding RNNs, we are investigating LSTMs and ConvLSTMs. This work will contribute to lower the errors on the receiver side and increasing the information throughput. Why is this important? You will be able to download your memes faster!
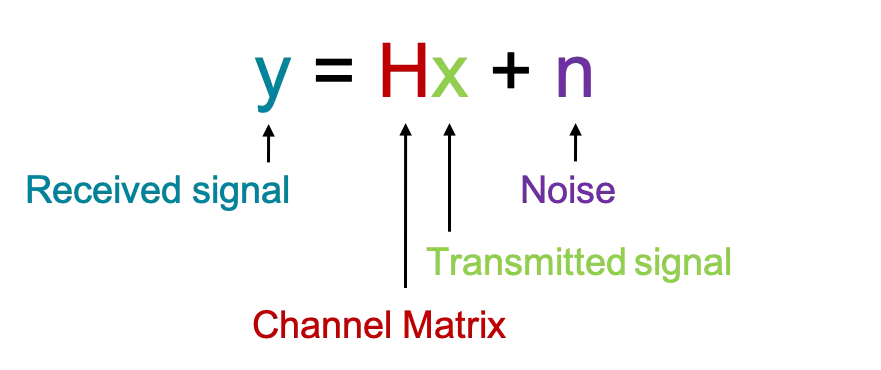
Link adaptation using Reinforcement Learning. We are developing an actor-critic RL algorithm that self-optimizes signal transmission. To this end, we are combining OpenAI Gym with Tensorflow and GNU Radio. Thanks to this work, 5G systems will adapt themselves to a changing environment resulting in more efficient information transmission. Eventually, this leads to an increase in speed and reliability while costs are decreasing.
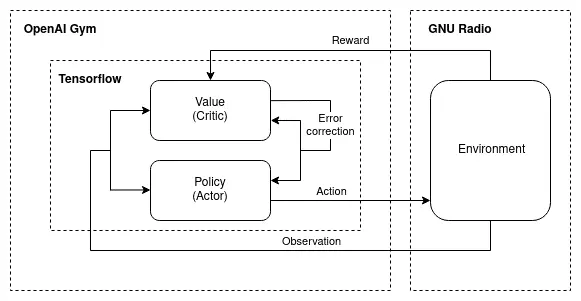
GNU Radio simulation environment. Last but not least, we are developing a simulation and testing environment. This allows us to carry out simulated and real-world experiments using intuitive flowgraphs, processing blocks, and software-defined radio (SDR) hardware. This work is crucial to validate our developed algorithms, as well as to build a system that is modular and reusable.
Beyond FunKI
FunKI will be finalized in May 2023. By then, we will have contributed to the scientific community proposing new advancements to the state of the art. In addition, we will have a deep understanding of how 5G can benefit from AI.
What comes next? How does June 2023 look like?
- We continue our research in wireless communications with an upcoming project, and further consolidate our position as experts in the 5G and AI community.
- We start working on a product in the 5G + AI space that highly impacts society. What about production lines so predictive they can prevent interruptions well before they occur? Or a communication system that facilitates vehicle-to-vehicle (V2V) communications? Or a communication device that enables now-unthinkable medical teleoperations?
It sounds interesting, right? Why don’t you join us in changing the future?