MTU Aero Engines Serial Number Identification With AI-Powered App
Faster manufacturing process, fewer errors and seamless traceability. We developed an AI-powered smartphone application for MTU Aero Engines that automatically reads turbine blade serial numbers with up to 99.65% accuracy.

The application has significantly improved our operational efficiency and user experience, with high user acceptance ensuring its sustainable use and long-term success.
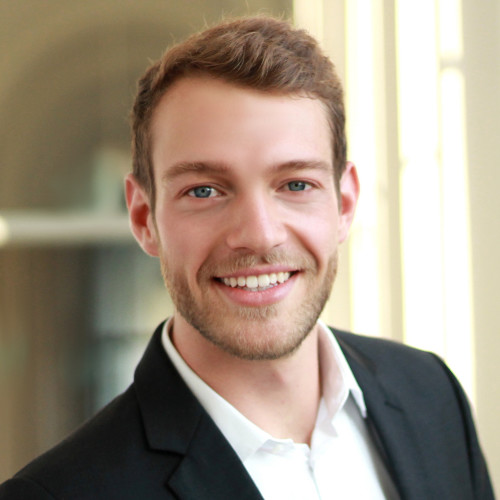
Our Final Result
Overview
Challenge
Solution
Technologies
Impact
Our Final Result
Our AI-driven serial number identification application significantly reduces the identification time per blade per step with 99.65% accuracy.
Streamlining the manufacturing process MTU Aero Engines:
- faster blade identification
- more reliable attribution
- seamless traceability and integration within workflow
- scalable to other use cases
Our application simplifies the tedious and error-prone turbine blade identification process. It has delivered significant efficiency gains, resulting in substantial annual cost savings in the low six-figure range, after covering all internal and external project-related expenses. Since the application is scalable to other use cases, potential future savings are even higher. Beyond labor savings, the application minimized human error, reduced strain on workers, and strengthened regulatory compliance through consistent data capture. It also frees up labor resources that can be used more efficiently in other manufacturing processes. Building on this success, the project transitioned from a proof of concept to full-scale production rollout, with planned enhancements such as barcode scanning and seamless ERP integration.

Looking for an
Innovation Partner?
Contact UsChallenge
Building an application to speed up the time-intensive and error-prone manual turbine blade identification process that requires high accuracy under challenging conditions.
Slow, Error-Prone, and Physically Demanding Identification
In turbine blade manufacturing, each blade must be repeatedly identified for traceability, quality control, and compliance. Workers have to use magnifying glasses to manually read tiny dot-peen serial numbers. The high number of required identification steps and strict regulatory demands makes turbine blade manufacturing very time-consuming. Additionally, manual data entry risks inaccuracies and fatigue among workers. The serial numbers that have to be identified in this process are hard to read, since they are minuscule and on reflective metal surfaces.
Ensuring High Accuracy under Real-World Conditions
MTU Aero Engines sought an efficient, accurate, and automated solution that can operate in their unique work environment. However, initial training data was limited, and the need for on-device inference demanded a multifaceted technical approach.
Solution
We developed an AI-powered smartphone application that captures and interprets serial numbers reliably, even in challenging lighting conditions. Synthetic training data enabled high accuracy of on-device inference.
All About MTU Aero Engines
Germany’s Leading Engine Manufacturer
MTU Aero Engines is an expert in the development, manufacturing, and maintenance of civil and military engines across all thrust and performance categories, as well as stationary industrial gas turbines. Their innovative propulsion systems, high-tech solutions, and comprehensive services enhance aviation efficiency, safety, and sustainability. In the field of civil maintenance, MTU Aero Engines Maintenance ranks among the top 3 global providers for civil aviation propulsion systems and industrial gas turbines.

Tackling the Challenge in a Four-Step Process
POC
The project began with an initial challenge of developing an AI-powered solution for turbine blade serial number identification using limited sample data. Also, we needed to find a solution that can operate in difficult lighting situations and perform on-device inference. The early prototype demonstrated the potential of the approach, despite initial low detection accuracy of only 20%.
Iteration
To overcome the initial limitations, the team generated synthetic training data using Blender and collected over 5000 real images to refine the machine learning model. This crucial step involved switching to a YOLO-based OCR architecture, which significantly improved the recognition capabilities. In this step, we also built a custom attachment for optimal lighting angles.
Testing
Rigorous testing was conducted until we ultimately achieved 99.65% accuracy in serial number identification. The team focused on optimizing the model for edge-based inference, ensuring efficient performance on smartphone devices while maintaining high precision. Based on user feedback from MTU Aero Engines workers, we additionally optimized the custom phone attachment to achieve the desired accuracy and improve usability.
Production Rollout
Successfully transitioning from proof of concept, the application was fully implemented into the manufacturing workflow at MTU Aero Engines. The production version includes planned enhancements such as barcode scanning and seamless ERP integration, setting the stage for broader application across different manufacturing processes.